The aim of this blog is to highlight the significant benefits of applying modelling and simulation (M&S) in the pharmaceutical industry without compromising product quality. The applications range from R&D to manufacturing decision-support tools being applicable to existing manufacturing processes or manufacturing processes under development. Incorporating M&S is the ultimate demonstration and documentation of the available process knowledge, which comprises the foundation of a pharmaceutical quality system – “Quality can not be tested into products; quality should be built-in or be by design [via knowledge].”
Introduction
Continuous chemical manufacturing such as the petroleum industry has historically gained enormous benefits from process systems engineering tools such as process modelling and simulation, optimizing real-time control, and optimal design. The pharmaceutical sector is largely governed by batch processing, where process implementations and changes are predominantly driven by empirical data or legacy procedures. In-silico (computational) tools have received increasing attention by the regulatory bodies (FDA, EMEA, etc.) due to the emergence of data analytics technologies including AI/ML in combination with technological advancements on process analytical technologies (PAT) as key enablers for enhanced quality by design, continuous manufacturing, and real-time release. Despite the obligations to continuous improvements to offer safe, effective, and affordable medicines, widespread exploration and implementation of in-silico tools are hindered due to regulatory uncertainty and scientific /technical complications [1].
Mechanistic Models versus Data-driven Models
Before diving into applications of M&S, it is important to frame the difference between mechanistic models and data-driven models. In this context, a mechanistic model is a systematic breakdown of a complex process into simpler physical phenomena (e.g., diffusion, reaction, heat conduction) based on the examination of observations, followed by additively constituting the phenomena into spatially resolved conservation balances. Ideally, each phenomenon can be studied and parameterized independently. On the contrary, a data-driven model is a “black box” input-output relation, established a data set of sufficient volume and quality (variance, structure, annotation, etc.).
Where mechanistic models dominate low data-volume applications (development, abnormal operation) due to strong extrapolation ability, the data-driven models dominate real-time production applications due to data volume and relative low batch-to-batch variance.
Mathematically, conservation laws in mechanistic models typically consist of time derivatives (dynamic systems) and /or spatial derivatives (change in position). If more than two different derivatives are involved, a partial differential equation is resulted, which typically is discretized numerically in common simulation software. Discretization methods are for example finite element method (FEM) or finite volume method (FVM).
Modelling & Simulation Applications in Pharmaceutical Product Lifecycle
M&S are used to some extent across all departments in pharmaceutical companies. Reconciliation of vials (discrete material balance), diffusion flow rate estimation during flow filter integrity testing, economic models, warehouse inventory tracking, and so on.
Figure 1 gives an overview of applications specifically related to the pharmaceutical product life cycle including an indication of the level of model fidelity involved in M&S. The first category is high-fidelity models that rely on fundamental molecular interactions according to quantum mechanics. The second category is medium fidelity models that cover classical micro to macro scale spatial conservation models. Finally, low-fidelity models cover macro-scale homogenized models that are fast and suited for industrial simulators.
This blog will focus on medium fidelity models marked with superscript “b” in Figure 1.
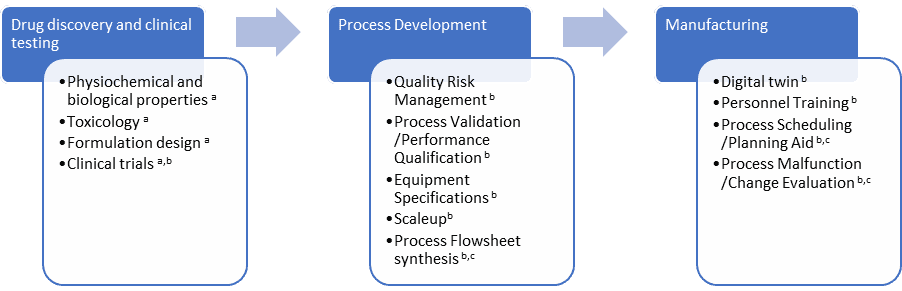
Modelling & Simulation During Drug Discovery and Clinical Testing
Pharmacokinetics describes the transport and action of a drug inside the organism where it is delivered. Due to the complex nature of living organisms, spatial variations must be accounted for in physical properties, which makes M&S utilizing FEM and FVM suited for conducting pharmacokinetic studies. One example is the COMSOL® application related to the pharmacokinetic study of a human eye [2].
Modelling & Simulation During Process Development
Medium fidelity M&S are particularly suited for Process Development.
Quality Risk Management (QRM)
Using process knowledge, M&S can be used to establish mathematical (models) relations between Process Parameters (PP’s), Material Attributes (MA’s) and Critical Quality Attributes (CQA’s) [3]. This enables (i) quantitative assessments, (ii) ranking PP’s and MA’s according to importance, (iii) objective criticality assessment to obtain Critical PP’s (CPP’s) and Critical MA’s (CMA’s), and (iv) solve for CPP and CMA subject to CQA constraints to obtain the design space. The use of M&S in QRM is illustrated in the examples in the following sections.
Process Validation (PV) /Performance Qualification (PQ)
These phases heavily rely on empirical evidence that the process performance is predictable within specified limits. M&S can aid in prioritization and prequalify test set points (e.g., design of experiments) to significantly shorten time and resources for test planning, materials, and execution, thereby ultimately shortening the time to market. M&S allows for subjective establishment of process limits during cycle development (e.g., autoclave, decontamination). See Figure 2. CFD has been demonstrated as a useful tool to evaluate impact of aeration parameters subject to individual loads, in order to optimize the decontamination cycle development and for example guide the placement of biological indicators to shorten test time [4]. Robust process design [5] and uncertainty and sensitivity analysis are essential tools for objectively identifying CPP limits and instrument maximum permissible error limits (MPE). The latter point is also relevant for the following point.
Equipment specification
M&S can be used to guide technology selection and to quantitatively evaluate vendor recommendations and options. Examples could be to establish maximum permissible error for critical measurement loops that dictates instrument design, or it could be determination of suitable working volumes based on scale-up (see Figure 2). After equipment installation, simulations can be performed on issued CAD-drawings of equipment to assist in qualification activities [6].
Scaleup
Promote scale-up due to reasonable ability to extrapolate and account for spatial variations. M&S is commonly applied in assisting in scaleup.
Process Flowsheet Synthesis
Conduct feasibility studies or refine performance evaluations on unit operation level for flowsheet synthesis, to reduce plant design uncertainty.
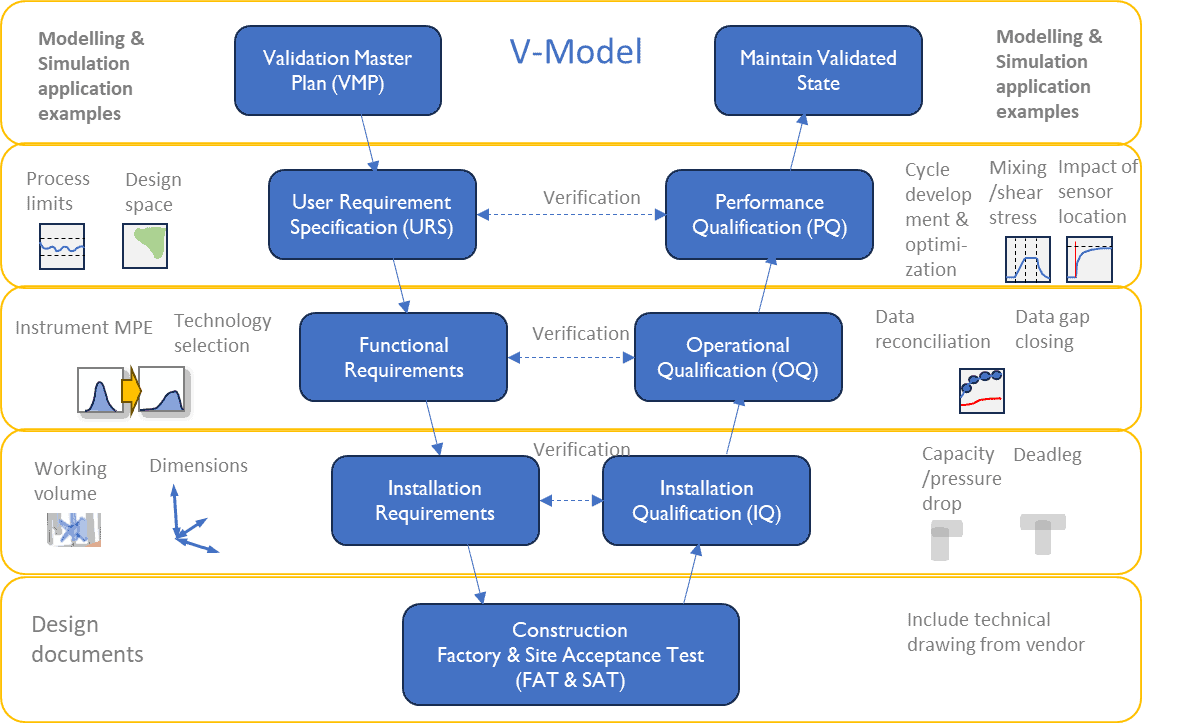
Modelling & Simulation During Manufacturing
Digital twin
Medium-fidelity models are in many cases solved slower than real time, thereby disqualifying them in digital twin applications. However, they can be used to generate the required data volume to train data-driven models, enabling cost efficient and low-risk proof-of-concept studies.
Personnel training
A systematic handover of process knowledge from the development unit to the manufacturing unit is often overlooked, thereby substantially increasing training time and risk of errors /deviations during early PV/PQ production runs. M&S allows personnel to digitally explore input (e.g., PP) and output (e.g., CQA) relations to overall strengthen the quality mindset and confidence in the manufacturing organization.
Process Malfunction /Change Evaluation
M&S enables rapid impact assessment of abnormal events /conditions and process changes. Hence, M&S work can be leveraged from the development phase. During the COVID-19 pandemic around 2020, many suppliers of essential raw materials failed to meet the demand of many pharmaceutical companies, which caused immense pressure on manufacturing sites. Having M&S integrated in the organization allows a rapid response by being able to prequalify available competing products in digital simulations. Similarly, retrofit designs and be evaluated prior to implementation, where M&S may aid in the impact assessment for change management. As simulation allows us to digitally test and measure parameters that are difficult to measure in the physical world, M&S may also have uses in assistance on solving and addressing reoccurring problems during manufacturing (e.g., corrective, and preventive actions CAPA’s).
Process Scheduling /Planning Aid
User-friendly applications can be derived from M&S that can predict processing times of manufacturing line bottlenecks based on product history knowledge and process inputs. See more information from the blog post on filter drying [7]. If process time is a CPP and its estimate exceeds the process limit, this information can be used to initiate timely proper actions.
Example: Quality Risk Management
The scientific literature offers several demonstrative cases of M&S-assisted Failure Mode, Effects and Criticality Analysis (FMECA) [8, 9]. The FMECA (or similar) is an essential tool in identifying, addressing, and communicating process risks [3].
Here we adopt an example inspired by example 1 in ICH Guideline Q11 [10] related to chemical synthesis of a drug substance (DS). The numbers are generated for the purpose of this illustration.
In one step of the synthesis, compound F is converted under reflux to G. The starting point consists of F and a water impurity W, which reacts irreversibly with F to I. The reaction schemes are:
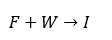
Assuming that compound I cannot be removed during purification steps and has impact on drug product quality, this impurity concentration is classified as a CQA. The simplest way to establish a quality system is to experimentally establish limits on process time (a CPP) and water concentration (a CPP) according to Column 5 in Figure 3. Based on limit on impurity of 0.0075[mol L-1], we can establish water concentration limit of 0.02[mol L-1] and process time limit of 3 h (see Figure 4).
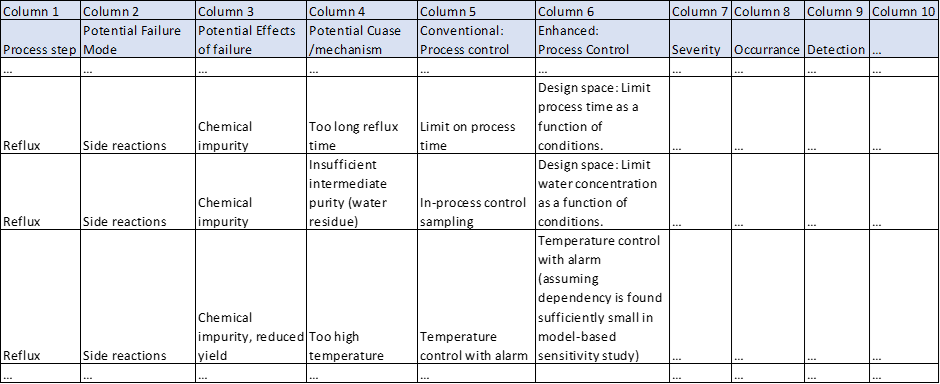
Instead, M&S is employed in a so-called enhanced approach. In this case, one has to establish a model:
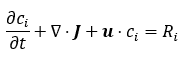
The above model describes time dependent concentration of compound i in 3D domain governed by diffusion (diffusion field J) and convective mixing (velocity field u
), and reaction ( Ri ). Such a problem is suited for FEM or FVM simulators. By neglecting diffusion and assuming ideal mixing, the model reduces to:

Reaction kinetics can be studied in controlled experiments to identify rate expressions with relevant parameters. In this case:
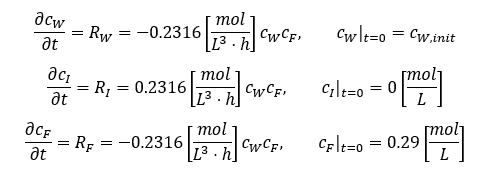
A simulation can be performed with various degrees of complexity. The design space can be obtained by solving the backward problem of identifying a water concentration and process time that yields an impurity concentration below 0.0075[mol L-1]. This is illustrated in Figure 4.
It is apparent that a significantly larger design space is available using the enhanced approach leading to higher flexibility when it comes to process changes, deviation handling, process improvements, etc., potentially reducing the number of scrapped batches. Working within the design space is not considered as a change [10]. Additional efforts are required for properly documenting the design space as illustrated in Figure 4. If the design space input dimension is more than two as in this example, visualization becomes challenging. Note also the severe impact imposed by poorly selected experimental conditions when selecting the process limits. A model can guide the selection of “close to limit” conditions for verification of the design space.
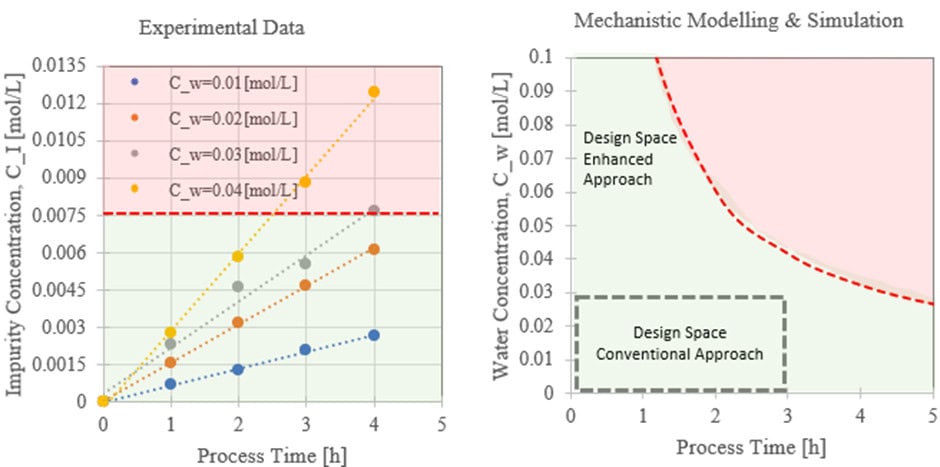
Why resolvent and conclusion
As highlighted in this BLOG, modelling and simulations (M&S) have numerous well-proven applications in the pharmaceutical industry, and some are yet to be discovered. We show that applying M&S, in contrast to shadowing the product quality focus, instead can be adopted to significantly strengthen your quality management system while at the same time permitting performance optimization.
resolvent offers a package of technical competences and a palette of computational tools to accommodate the customers’ needs in the fields of design, simulations, and digitalization. The offered core values are the following:
- optimization of products and processes,
- reduce time to market,
- multiply your experts,
- democratization, and
- flexible engineering resource.
The broad portfolio of project engagements in various industrial sectors qualifies us as a potential partner in formulating a simulation strategy in your organization.
Changelog
Version 2.0, Date 10-Oct-2023, Initials TB, Minor corrections.
Version 1.0, Date 29-Sep-2023, Initials TB, New post.
Literature
[1] “Guidance for Industry PAT — A Framework for Innovative Pharmaceutical Development, manufacturing, and Quality Assurance” Pharmaceutical CGMPs, September 2004, U.S. HHSW, FDA, CDER, CVM, ORA.
[2] https://www.comsol.com/paper/virtual-pharmacokinetic-model-of-the-human-eye-18101
[3] ICH Guideline Q9 on quality risk management – Step 5. European Medicines Agency. January 2006.
[4] Nayan, N.; Akay, H.U.; Walsh, M.R.; Bell, W.V.; Troyer, G.L.; Dukes, R.E.; Mohan, P. “CFD modeling of pharmaceutical isolators with experimental verification of airflow”. PDA J Pharm Sci Technol, 61(4) (2007):237-254.
[5] Xie, X., Schenkendorf, R. “Robust Process Design in Pharmaceutical Manufacturing under Batch-to-Batch Variation”. Processes, 7, 2019, 509. doi:10.3390/pr7080509.
[6] EudraLex Volume 4, EU Guidelines for Good Manufacturing Practice for Medicinal Products for Human and Veterinary Use- Annex 15: Qualification and Validation. Brussels, 30 March 2015.
[7] https://resolvent.com/model-based-drying-time-prediction-in-pharmaceutical-api-production/
[8] Rantanen, J., Khinast, J. “The Future of Pharmaceutical Manufacturing Sciences”, Journal of Pharmaceutical Sciences, 104, 2015, p. 3612-3638.
[9] Stocker, E., Toschkoff, G., Sacher, S., Khinast, J.G., “Use of mechanistic simulations as a quantitative risk-ranking tool within the quality by design framework”, International Journal of Pharmaceutics, 475 (2014): 245-255.
[10] ICH guideline Q11 on development and manufacture of drug substances (chemical entities and biotechnological/biological entities) – Step 5. European Medicines Agency. November 2012.